|
|
Thanks for subscribing, and welcome to the 1st edition of AI for Trust, Safety and Fraud, your monthly guide to the latest insights on machine learning for trust, safety, fraud detection, and risk management. My goal with this newsletter is to share valuable knowledge, updates, and resources to help you stay informed and empowered in this rapidly evolving field. In this edition of our newsletter, we'll navigate through three main sections. We'll first delve into Current Affairs, exploring some latest news in fraud and security. Then, we'll revisit some of the past insights from the blogposts - 'Fraud Detection Systems 101 and Beyond'. And finally, we'll shine the spotlight on a recent research piece in the field of mobile social network fraud detection. I will always be looking for ways to improve, so don't hesitate to share your feedback or suggestions. This would help in ensuring that you continue getting valuable content that serves your interests. Let's dive in!
| |
1. Current Affairs Payments Fraud and Control Survey - J.P. Morgan The latest (2023) Payments Fraud and Control Survey that is sponsored by J.P. Morgan for over 15 years is out, and following are the key insights:  - Payments fraud is still a serious threat for every organization. The share of businesses that report commercial card fraud has increased by 10 percentage points since 2021.
- Over half of organizations with annual revenue of less than $1 billion were unable to recover funds lost due to payment fraud attacks.
- Fraudsters continue to impersonate employees and vendors through sophisticated business email compromise (BEC) schemes that are the root cause of most reported fraud cases.
- Checks continue to be the payment method most vulnerable to fraud. Still, three of our four organizations that use checks plan to keep using checks.
- Business Email Compromises (BEC) scams are still highly prevalent and are the root cause of payments fraud at a majority of organizations. 71% of companies were victims of payments fraud via email in 2022. Payment methods often used during BEC included wires, (45% respondents) and ACH debits.
| |
The meta-owned WhatsApp app is ramping up to provide several privacy and safety tools to proactively weed out bad actors from the platform. This month, WhatsApp introduced features to tackle spam calls which are highly prevalent in India - Tech Crunch. India is the biggest market for WhatsApp with over 500 million users. Based on user's reports, these spam calls promote fraudulent job offers and originate from international codes from countries including Ethiopia (+251), Indonesia (+62), Kenya (+254), Malaysia (+60) and Vietnam (+84), among others. 2022 U.S Spam and Scam - Truecaller Continuing the discussion of spam calls, some insights from 2022 U.S Spam and Scam Report published by Truecaller (yeah, not really a current news in 2023): Phone scams are relentless and the numbers don’t lie. A staggering $39.5 billion USD (extrapolation based on results of survey on ~2000 adults) was lost to phone scams in America over the past 12 months. Americans received an average of 31 spam calls in a month, which was higher than the year before. Common reasons of these calls? Vehicle warranties, medicare, money owed for tax and bundle offers. | |
2. Blogposts: Fraud Detection 101 and BeyondIn case you missed, my blogpost series on 'Fraud Detection 101 and Beyond', following are 2-line summaries of the three articles I have written in the past. Each article delves into essential aspects of fraud detection and risk management. 1. Types of Fraud and Abuse - In this article, I explore various forms of fraud and abuse prevalent in today's digital landscape. By understanding the different types of fraud, such as credit card fraud, identity theft, fake news, account takeover etc., we can better design and implement effective detection and prevention strategies. 2. Traditional Detection Framework - This piece focuses on traditional fraud detection frameworks, which have been the backbone of many anti-fraud efforts. I discuss the strengths and weaknesses of rule-based systems, statistical models, and other conventional approaches, highlighting the importance of continuous adaptation to keep up with evolving threats. 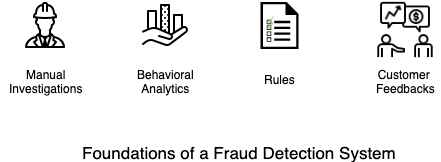 3. Fraud Detection Frameworks - In this article, I provide general frameworks to approach the new problem in fraud detection depending on the data and/or label availability. I cover various techniques, including supervised and unsupervised learning, reputation scores, consortium and more, emphasizing their potential in the field of fraud prevention and risk management. | |
3. Spotlight Research
Cost Sensitive GNN-based Imbalanced Learning for Mobile Social Network Fraud Detection - Xinxin Hu et al. @ IEEE Transactions on Computational Social Systems, 2022 Published in IEEE Transactions on Computational Social Systems on Dec 2022, this paper by Xinxin Hu et al., proposes a cost sensitive graph neural network (GNN) based imbalanced learning method for fraud detection in mobile social networks. Let's dive in:  What is mobile social network fraud detection? Mobile social network fraud detection involves identifying misuse of mobile services, such as spam calls or texts, to extract money. In 2022, this type of fraud resulted in an estimated loss of 39.5 billion USD. What is imbalance problem? In fraud scenarios, fraudulent users make up a small fraction of all users, resulting in imbalanced data. In the context of graphs, fraudulent nodes tend to have a large number of legitimate nodes in their vicinity, negatively affecting model predictions. Why traditional imbalance data handling would not work? Traditional methods, such as oversampling fraud events or undersampling legitimate ones, have drawbacks. Oversampling may lead to overfitting, while undersampling could result in information loss. Class weighting, a third method, gives different weights to fraudulent and legitimate events. This paper combines sampling and class weighting to handle imbalance. How authors addressed it? To improve node classification in imbalanced graphs, the authors proposed two strategies: RL-based neighbor sampling: Reinforcement Learning (RL) is used to balance the fraudulent and legitimate population by determining how many similar neighbors should be sampled for each node. (middle plot in the figure above) Cost-sensitive learning: After slightly balancing sub-graphs via neighbor sampling, a cost-sensitive loss function is used. This function imposes a higher penalty for misclassifying fraud than for misclassifying legitimate nodes. This is achieved by transforming the output GNN embeddings using a learnable cost-sensitive matrix, which emphasizes the different penalties for different types of misclassification. (right plot in the figure above) What experiments were performed to prove that approach works? The authors conducted experiments on two real-world, imbalanced telecom fraud datasets, having imbalance ratio of 0.47 and 0.08. They compared multiple GNN classification methods, including general GNNs, those optimized for fraud detection, and ones tuned for imbalanced data, against their proposed approach. The results demonstrated that their method outperformed all other compared approaches on both datasets. | |
Next month, 'AI for Trust, Safety and Fraud' will bring you more thought-provoking articles, industry news, and practical resources. Stay tuned! The newsletter includes author's interpretation of news and research works, and can have errors. Please feel free to share feedbacks, errors or questions, if any. | |
|
|
|